VoP: Text-Video Co-operative Prompt Tuning for Cross-Modal Retrieval
Published in Proceedings of the IEEE/CVF Conference on Computer Vision and Pattern Recognition 2023 (CVPR 2023)
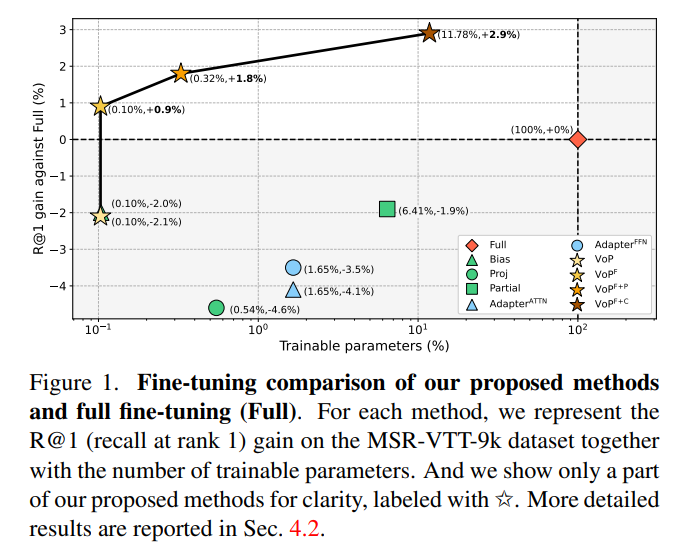
In this work, we propose the VoP: Text-Video Co-operative Prompt Tuning for efficient tuning on the text-video retrieval task. The proposed VoP is an end-to-end framework with both video & text prompts introducing, which can be regarded as a powerful baseline with only 0.1% trainable parameters. Further, based on the spatio-temporal characteristics of videos, we develop three novel video prompt mechanisms to improve the performance with different scales of trainable parameters. The basic idea of the VoP enhancement is to model the frame position, frame context, and layer function with specific trainable prompts, respectively. Extensive experiments show that compared to full finetuning, the enhanced VoP achieves a 1.4% average R@1 gain across five text-video retrieval benchmarks with 6× less parameter overhead.